Nobel Prize in Physics for foundational work on deep learning with artificial neural networks
8 October, 2024
The Royal Swedish Academy of Sciences has decided to award the 2024 #NobelPrize in Physics to John J. Hopfield and Geoffrey E. Hinton ‘for fundamental discoveries and inventions enabling machine learning with artificial neural networks’. Hinton ‘for fundamental discoveries and inventions enabling machine learning with artificial neural networks’.
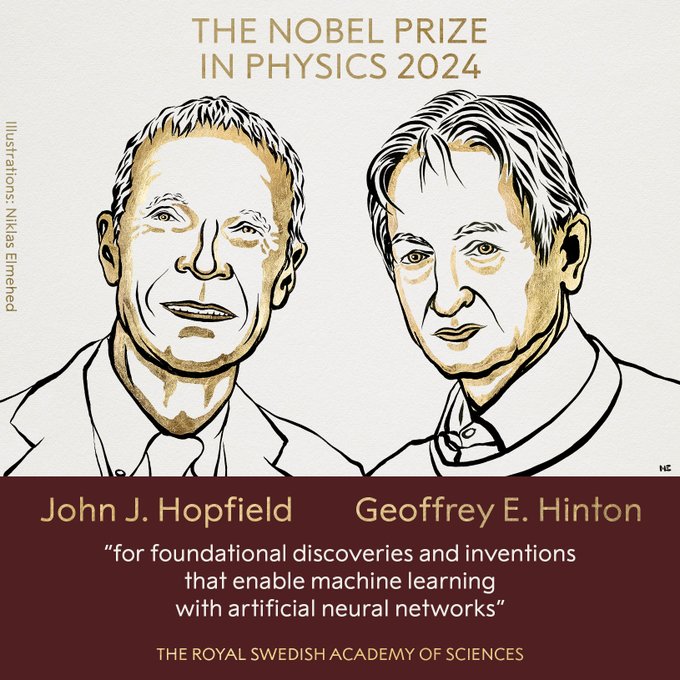
Fellow journalist Antonio Martínez Ron has been quick and has published the following news in Diario.es
John J. Hopfield’s most cited work:
The study explores the computational properties that can arise from large numbers of interconnected single neurons. It focuses on investigating how collective behaviour in such neural networks can give rise to useful computational capabilities, similar to how collective phenomena in physical systems can give rise to interesting properties. Full text
The most frequently cited article by Geoffrey E. Hinton:
The article discusses the use of convolutional neural networks (CNNs) for image classification, specifically on the ImageNet dataset, which contains over 15 million high-resolution labelled images. The authors trained one of the largest CNNs to date on subsets of the ImageNet dataset used in the ImageNet Large-Scale Visual Recognition Challenge (ILSVRC). Full text
Science Media Center España has asked us for a simple quote to help journalists understand this news.
Our director Francisco Herrera tells us: ‘In the first half of the 1980s there was a revival of artificial intelligence (AI), after the so-called AI reversal of the 1970s, thanks to the important developments in the field of artificial neural networks led by John Hopfiled and Geoffrey E. Hinton. Hinton.
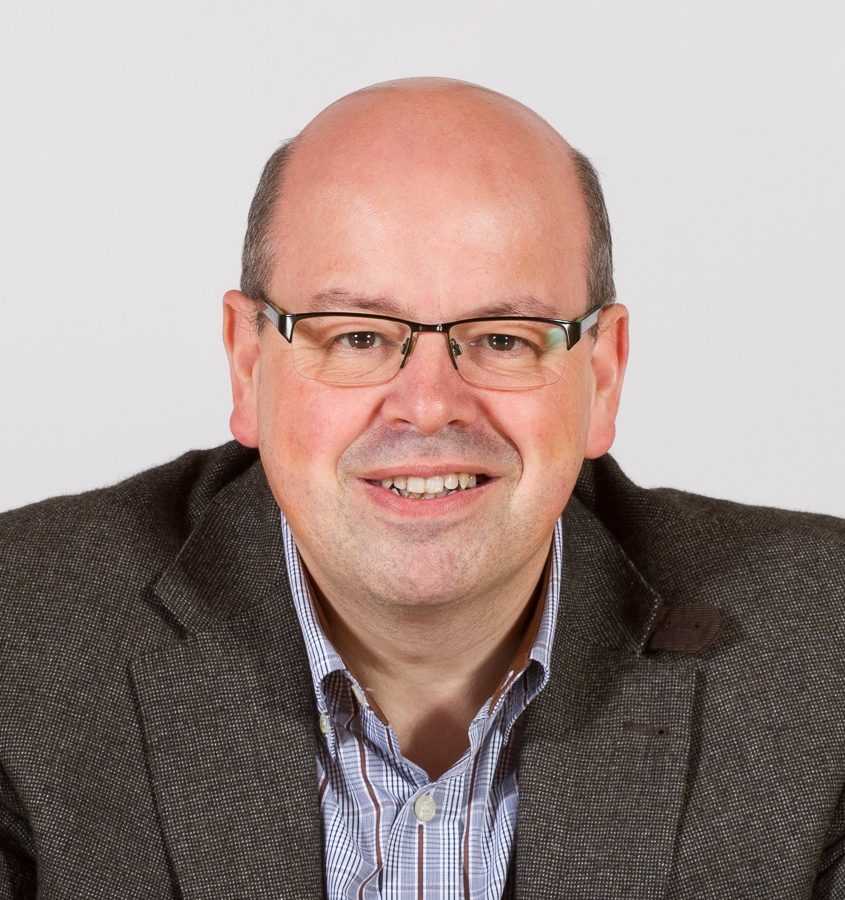
Hopfiled in 1982 connected the biological aspects of nervous systems with the computational domain. In his paper entitled ‘Neural networks and physical systems with emergent collective computational abilities’ (PNAS, 1982) he notes ‘Computational properties useful for biological organisms or for the construction of computers can emerge as collective properties of systems having a large number of simple equivalent components (or neurons). The physical meaning of addressable content memory is described by an appropriate phase space flow of the state of a system.’ With his proposal he defines the so-called Hopfield networks, a type of recurrent artificial neural network, which are used as associative memory systems with binary units, which converge in their learning process, and which have applications in different fields such as image processing, speech processing, among others.
Geoffrey E. Hinton was the father of the training and learning model of multi-layer meeting models (the one-layer model was the so-called perceptron of the seventies), called backpropagation. It is a supervised learning method that adjusts the weights of connections in a neural network to minimise the error between the actual output and the desired output. Backpropagation has been crucial to the development of deep learning, because it allows deep neural networks to be trained efficiently, adjusting weights systematically to minimise error, helps neural networks learn internal representations of data, which improves their ability to generalise to new data, and opened the door to developments in deep learning, image processing, speech, text … It is the basis of what is now the hatching of generative AI.
These results from the first half of the 1980s laid the foundation stones for the development of the following 40 years that have led to the current emergence of artificial intelligence and deep learning, which is based on these results that sought to emulate the functioning of the human neuronal system’.
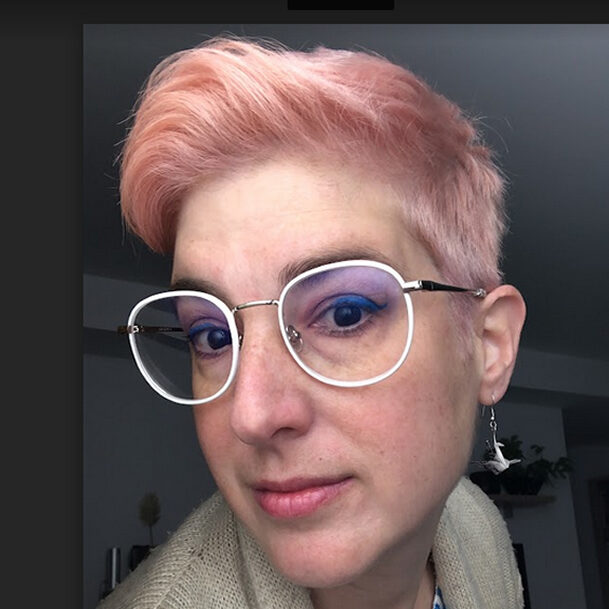
For her part, our colleague Rocío Romero points out that: ‘It must have been very difficult to choose which researchers were awarded this year’s Nobel Prize in Physics for the foundational discoveries and inventions that enable machine learning with artificial neural networks. Research in the area began in the 1950s and after several periods of greater and lesser scientific development, it has come to the present day with renewed vigour. Both John Hopfield and Geoffrey Hinton stand out for designing different types of artificial neural networks that have given rise to the creation of today’s artificial intelligence tools that allow both the detection of cancers in early stages and the generation of realistic artificial text. It is, in turn, surprising news given that there is no Nobel prize for computer science, highlighting the fact that multidisciplinary work is clearly the future of cutting-edge research’.