AI tool developed to anticipate metabolic risks in obese children
13 December, 2024
The work is part of the EprObes (‘Early Prevention of Obesity’) project coordinated by the CIBER and in which UGR researchers participate, including 3 from the DaSCI institute. It has been published in the journal ‘Artificial Intelligence in Medicine’.
The AI model uses clinical and epigenetic data to personalise the prediction of metabolic risks, allowing early interventions that could reduce the comorbidities of obesity and healthcare costs.
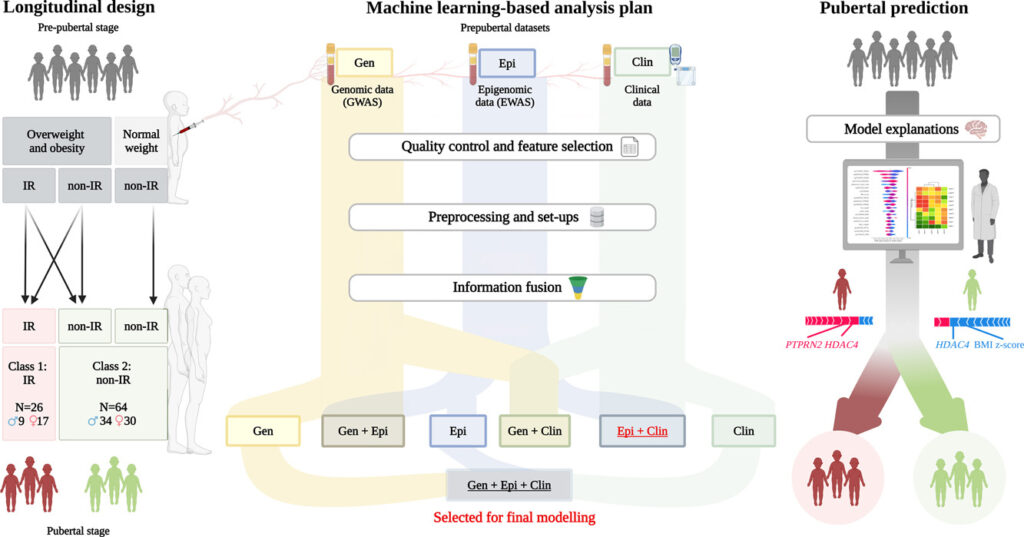
Several teams from the Centro de Investigación Biomédica en Red (CIBER) at the University of Granada (UGR) have developed an Artificial Intelligence (AI) explainable model to predict the risk of metabolic alterations in children with obesity. This study has been carried out by researchers led by Mercedes Gil-Campos and Concepción M. Aguilera García of the Physiopathology of Obesity and Nutrition area of the CIBER (CIBEROBN), in the Department of Biochemistry and Molecular Biology II, the José Mataix Institute of Nutrition and Food Technology (CIBM), the Institute of Biosanitary Research (ibs.GRANADA) and the researchers Farael Alcalá, Jesús Alcalá and María Arteaga of the Andalusian Inter-University Institute in Data Science and Computational Intelligence (DaSCI).
The multidisciplinary team has developed an innovative explainable Artificial Intelligence (AI) model to predict the risk of insulin resistance in children with obesity. This breakthrough represents an important step towards personalised medicine and early detection of metabolic risks, with the aim of preventing long-term complications associated with childhood obesity.
Insulin resistance is a key factor linking obesity to an increased risk of cardiovascular disease later in life. Puberty has been identified as a critical time after which this condition becomes more difficult to reverse. Therefore, anticipating its onset is essential to implement effective interventions to reduce comorbidities and associated costs.
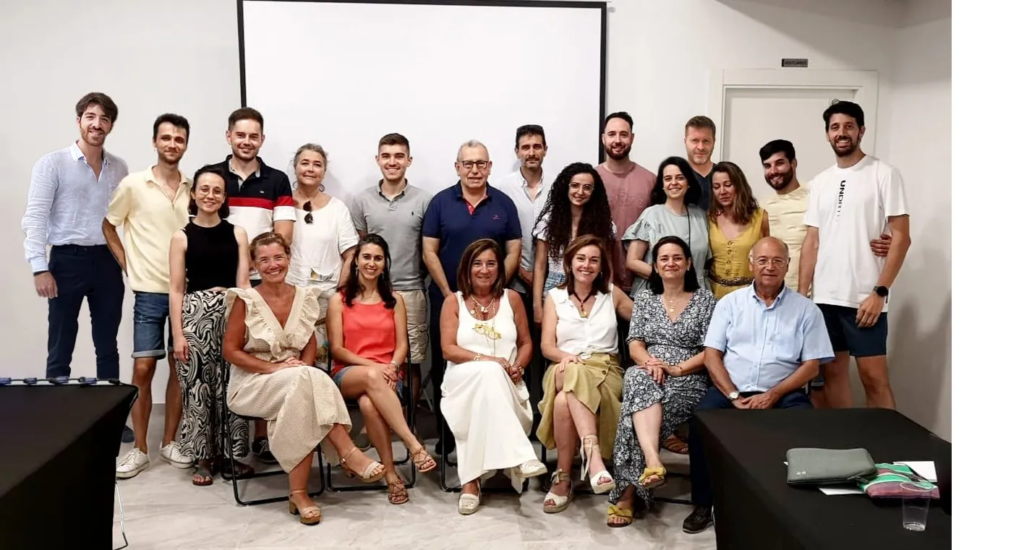
A multi-omics and explainable AI-based approach
The study, published in the journal Artificial Intelligence in Medicine, was conducted in a longitudinal cohort of 90 prepubertal boys and girls. The AI model combines traditional clinical data, such as body mass index (BMI) and leptin/adiponectin ratio, with novel epigenetic biomarkers in key genes (HDAC4, PTPRN2, MATN2, RASGRF1 and EBF1). In addition, it uses explanations generated by SHapley Additive exPlanations (SHAP) to provide healthcare professionals with a clear understanding of how the model works and the impact of the variables in each prediction.
The system has demonstrated a high predictive ability, with an area under the curve (AUC) and a geometric mean (G-mean) of 0.92. These results underline the value of integrating multi-omics data and explainable AI methodologies to address complex problems in clinical settings.
Clinical impact and international collaboration
This breakthrough could revolutionise the way metabolic risks are detected and managed in hospitals. By allowing pharmacological interventions or lifestyle adjustments at an early stage, the model not only improves patients’ prognosis, but could also significantly reduce public health costs.
The project has been funded by the Instituto de Salud Carlos III and the European programme EprObes (Preventing lifetime obesity by early risk-factor identification, prognosis and intervention), which addresses obesity as a serious public health problem from a multidisciplinary approach. In addition to the University of Granada, clinical groups from the IDIS of Santiago de Compostela, the IIS of Aragon and other national and international centres have participated.
Towards personalised medicine
The use of bioinformatics technologies and artificial intelligence allows progress to be made towards more personalised and effective interventions. As the researcher Álvaro Torres, of the CIBEROBN, points out, ‘this combination of data not only allows precise predictions, but also a deeper understanding of the decision-making process of the model, which facilitates its clinical application’.
This work represents an example of how collaboration between basic and clinical research, as well as the use of advanced technologies, can transform the prevention and treatment of metabolic diseases from the earliest stages of life.
The CIBER Consortium (Centro de Investigación Biomédica en Red) depends on the Carlos III Health Institute – Ministry of Science, Innovation and Universities and aims to promote research of excellence in biomedicine and health sciences carried out in the National Health System and in the Science and Technology System. It currently has 513 research groups belonging to 105 consortium institutions in Spain.
Article reference
Torres-Martos, Á., Anguita-Ruiz, A., Bustos-Aibar, M., et al. (2024). Multiomics and eXplainable artificial intelligence for decision support in insulin resistance early diagnosis: A pediatric population-based longitudinal study. Artificial Intelligence in Medicine, 156, 102962. https://doi.org/10.1016/j.artmed.2024.102962