Nobel Prizes in Physics and Chemistry for applied Artificial Intelligence tools
4 November, 2024
This year, the Royal Swedish Academy of Sciences announced, on the one hand, the Nobel Prize in Physics ex aequo for two artificial intelligence (AI) researchers, John Hopfield (Princeton University) and Geoffrey E. Hinton (University of Toronto) and, on the other hand, the 2024 Nobel Prize in Chemistry shared by David Baker (University of Washington) for computational protein design and, jointly, Demis Hassabis and John Jumper (Google DeepMind) for protein structure prediction.
Nobel Prize in Physics
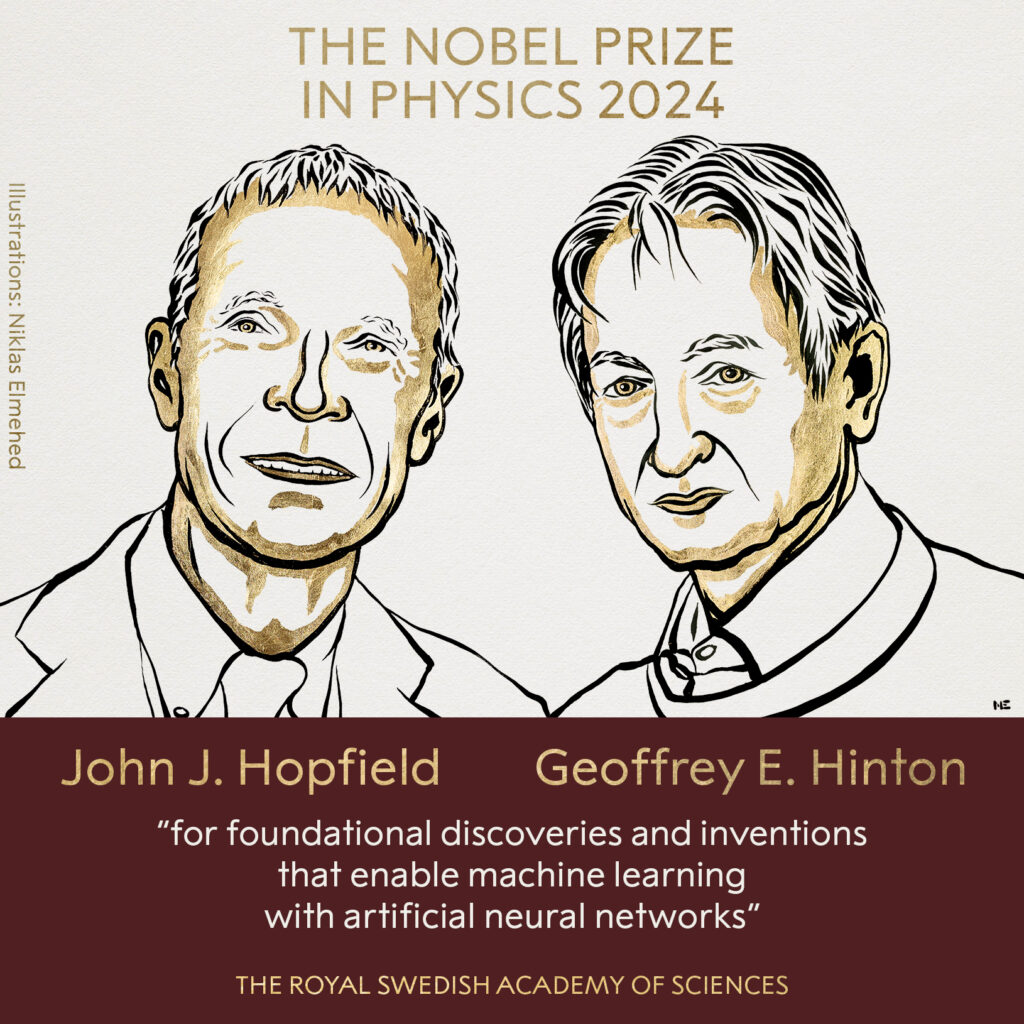
In its announcement, the Academy notes that ‘This year’s laureates used tools from physics to build methods that helped lay the foundations for today’s powerful machine learning’. Hopfield created a structure that can store and reconstruct information, Hopfield networks, in 1982, and Hinton invented the mechanism of learning systems with multiple layers of artificial neurons (backpropagation), a method that allows properties in data to be discovered independently and is of paramount importance for learning the large artificial neural network models used today.
What do these advances and the results of these researchers mean in the current artificial intelligence scenario?
The 1970s and early 1980s were the so-called ‘AI winter’. Those at the time were not living up to the high expectations and investments. It had been shown that the neural models of the time (the perceptron, with a single layer of neurons) could not solve complex problems. Symbolic models such as expert systems had a very limited practical scope.
Hopfield’s and Hinton’s results independently made discoveries that were arguably instrumental in a revival of connectionist AI, models with multiple layers of artificial neurons that help computers learn more than the human brain does, providing the basis for the development of today’s AI, with deep learning, the name given to large models of multiple layers of artificial neurons (deep learning), as the core technology.
The award recognises the growing importance of artificial intelligence in people’s lives and work. Artificial neural networks, thanks to their ability to interpret large amounts of data, play a crucial role in scientific research, including physics, where they are used to design new materials, process data from particle accelerators and study the universe. DeepMind’s AI system, GNoME, has already been used to predict the structures of 2.2 million new materials. Among them, more than 700 have been created in the lab and are currently being tested.
Nobel Prize in Chemistry

The Academy’s press release explains that this year’s prize ‘is about proteins’. On the one hand, the award goes to David Baker who ‘has achieved the almost impossible feat of constructing entirely new types of proteins’. On the other hand, Demis Hassabis and John Jumper have ‘developed an AI model to solve a 50-year-old problem: predicting the complex structures of proteins’.
These advances have enabled researchers in the fields of biochemistry, biology and medicine to make great strides in recent years. To get an idea of the potential of these discoveries, we have to remember that just over a decade ago it took several years to obtain the structure of a protein using the X-ray crystallography technique, whereas today, thanks to the work of these researchers, the structure of a protein can be predicted using Alphafold in just a couple of minutes.
On a historical note, the first version of AlphaFold won first place in the overall ranking of the 13th edition (2018) of the CASP (Critical Assessment of Techniques for Protein Structure Prediction) competition. Alphafold managed to predict structures for which there were no previous models, i.e. it had no information on similar proteins to rely on, making this work much more difficult and thus giving a leap in quality in protein structure prediction.
But what are these innovative tools that have marked a turning point in research?
Both RFdiffusion (created by David Baker’s research group) and Alphafold (created by Demis Hassabis and John Jumper as part of Google DeepMind) are tools that fall into the category of Artificial Intelligence. On the one hand, RFdiffusion was created for de novo protein design, i.e. generating proteins with specific structural and/or functional properties. This is achieved through the use of diffusion models, the same ones used by Stable Diffusion to generate realistic images. On the other hand, Alphafold is based on a particular type of artificial neural networks, called Transformers. This particular type of artificial neural networks developed in 2017 by Google researchers have revolutionised the world and are part of the reason why AI is so fashionable today. You may be familiar with them, given that other more mundane tools such as ChatGPT also use this technology.
Artificial Intelligence as a fundamental tool for scientific progress
Among the major challenges in the use of AI are advances in medicine and in the environment and climate change. In the first case, advances in medicine include, for example, the early detection of certain types of cancer. In terms of the environment and climate change, models such as ClimateGPT and NeuralGCM stand out for their ability to study climate change and make accurate weather predictions much more efficiently than conventional models.
Currently, the paradigm of generative AI, image and language creation, with large language models (LLMs) as its main exponent (among them the well-known ChatGPT), stands out. LLMs, trained on large amounts of textual data, can process and generate natural language for tasks such as translation and text generation. These models are based on the aforementioned Transformers architecture, which uses attention mechanisms to handle long-term dependencies in text sequences, overcoming the limitations of previous models.
Geoffrey Hinton, after years working with Google, has retired to focus on the ethical aspects of AI. In a recent article in Science (May 2024) ‘Managing AI’s extreme risks amid rapid progress’, he addresses the risks of rapid AI advancement, such as malicious use and potential loss of human control, proposing a comprehensive plan that combines research and proactive governance. The development of trustworthy AI and human-centred AI is the big goal of this decade. The regulations and governance proposals of the European Commission and the United Nations give us confidence in moving towards safe, governable and trustworthy AI.
Hopfield and Hinton’s findings fuelled the AI boom, initiating an ‘AI spring’ that has led to a moment of anticipation unprecedented in the last 40 years. Pioneering researchers advanced neural networks, and other scientists built on these studies to develop today’s AI models. This recognition highlights the relevance of AI today thanks to decades of research and the collective efforts of the scientific community. AI is transforming diverse areas of human life, from medicine to all areas of technology and science.
Original text by:
Francisco Herrera – Professor of Computer Science and Artificial Intelligence, Director of the Interuniversity Research Institute DaSCI, University of Granada, Full Academician of the Royal Academy of Engineering.
Rocio Celeste Romero Zaliz – Associate Professor at the Department of Computer Science and Artificial Intelligence, University of Granada, Deputy Director of Research, Transfer and Teaching at the Information and Communication Technologies Research Centre.
Some related articles in The Conversation:
- Los Nobel de este año: qué tiene que ver la física con la inteligencia artificial
- Nobel de Física para los pioneros de las redes neuronales que sentaron las bases de la IA
- Cuando la biología salta del laboratorio al ordenador… y al Nobel
- Nobel de Química a la estructura de las proteínas una vez más… ¿la última?