TripAdvisor Monuments
We present TripAdvisor Monuments (TripM), three new sets of reviews from TripAdvisor referred to the most popular monuments in Spain.
Part I. Datasets description
The Alhambra data set consists of a total of 7,217 opinions, of which 6,781 are labelled positive, 143 negative and 293 neutral. The Mezquita de Córdoba dataset is made up of a total of 3,525 opinions, of which 3,453 are labelled positive, 17 as negative opinions and 55 as neutral opinions. The Sagrada Familia dataset is made up of a total of 43,540 opinions, of which 41,163 are labelled as positive, 554 as negative opinions and 1,818 as neutral opinions.
With regard to the Italian monuments, the Grand Canal dataset is made up of a total of 14,484 opinions, of which 13,832 are labelled as positive, 104 as negative and 548 as neutral reviews. The Trevi Fountain dataset consists of a total of 25,391 opinions, of which 19,515 are labelled positive, 2,513 as negative reviews and 3,363 as neutral reviews. The Pantheon data set consists of a total of 24,829 opinions, of which 23,635 are labelled as positive, 107 as negative and 1,087 as neutral reviews.
The most relevant characteristics of each of the Spanish monuments datasets are shown below.
- User name: The name of the user in TripAdvisor.
- User location: The location of the user.
- User information: The total number of reviews, attraction re- views and helpful votes of the user.
- Review title: A main title of the text.
- TripAdvisor bubble rating: The writer’s overall qualification of the review. It is expressed as a bubble scale from 1 to 5 (from Terrible to Excellent ).
- Review date: The reviewing time.
- Review: The text of the opinion.
The characteristics of each of the Itanian monuments datasets are shown below.
- Review: The text of the opinion.
- TripAdvisor bubble rating: The writer’s overall qualification of the review. It is expressed as a bubble scale from 1 to 5 (from Terrible to Excellent ).
The following table shows the main characteristics of the data sets.
Reviews | Pos. reviews | Neg. reviews | Neu. Reviews | Words | Sentences | Study | |
Alhambra | 7217 | 6781 | 143 | 293 | 676398 | 35867 | (1), (2), (3) |
Grand canal | 14484 | 13832 | 104 | 548 | 539465 | 47943 | (1) |
Mezquita de Córdoba | 3525 | 3453 | 17 | 55 | 217640 | 13083 | (1), (3) |
Pantheon | 24829 | 23635 | 107 | 1087 | 774765 | 76720 | (1) |
Sagrada Familia | 43540 | 41163 | 554 | 1818 | 2220719 | 136181 | (1), (2) |
Trevi Fountain | 25391 | 19515 | 2513 | 3363 | 764998 | 70407 | (1) |
Part II. Studies/papers published using these datasets
These datasets have been used as a source of data for many sentiment analysis studies in the domain of cultural monuments. In our case, these datasets have been used for the following studies, among others:
(1) To study the viability of TripAdvisor as a source for cultural monuments studies.
In this scenario, we also aim at addressing the inconsistency’s problem detected. Due to the high cost of developing a Sentiment Analysis Method (SAM), we proposed to apply several of-the-shelf SAMs in TripM. We find out that the correlation between SAMs and users polarities is very low. One of the causes of these inconsistencies is the domain adaptation problem. In addition, we detect another possible reason and it is related with the variability of polarities in a same document. When someone writes a review and evaluates it with a scale of 1-5 (as in TripAdvisor), it does not express the same polarity for all the sentences of the same document. For example, if the experience is valued with a 5 (Excellent), we can find sentences with a negative connotation, and vice versa. More information is available in the related publication:
Valdivia, Ana & Hrabova, Emiliya & Chaturvedi, Iti & Luzon, Maria & Troiano, Luigi & Cambria, Erik & Herrera, Francisco. (2019). Inconsistencies on TripAdvisor Reviews: a Unified Index between Users and Sentiment Analysis Methods. Neurocomputing. 10.1016/j.neucom.2018.09.096.
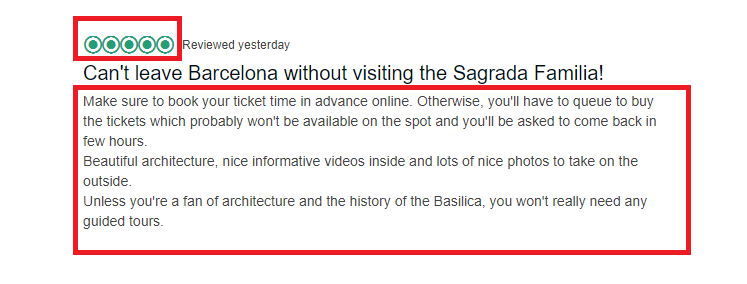
(2) To improve the performance of polarity classification methods.
For this purpose, we propose focusing on the detection of neutrality and treat it as the concept of noise in classical classification. In this way, we will obtain more precise methods when detecting positive and negative polarities. More information is available in the related publication:
Valdivia, Ana & Luzon, Maria & Cambria, Erik & Herrera, Francisco. (2018). Consensus Vote Models for Detecting and Filtering Neutrality in Sentiment Analysis. Information Fusion. 44. 10.1016/j.inffus.2018.03.007.
(3) Opinion summarization.
We believe that it is of utmost importance to create techniques that are capable of summarizing automatically the substantial content of opinions. These methods can help the decision-making processes of cultural organizations, detecting clearly those aspects that people like and those that need to be improved. More information is available in the related publication:
Valdivia, Ana & Martínez-Cámara, Eugenio & Chaturvedi, Iti & Luzon, Maria & Cambria, Erik & Ong, Yew & Herrera, Francisco. (2018). What do people think about this monument? Understanding negative reviews via deep learning, clustering and descriptive rules. Journal of Ambient Intelligence and Humanized Computing. 10.1007/s12652-018-1150-3.
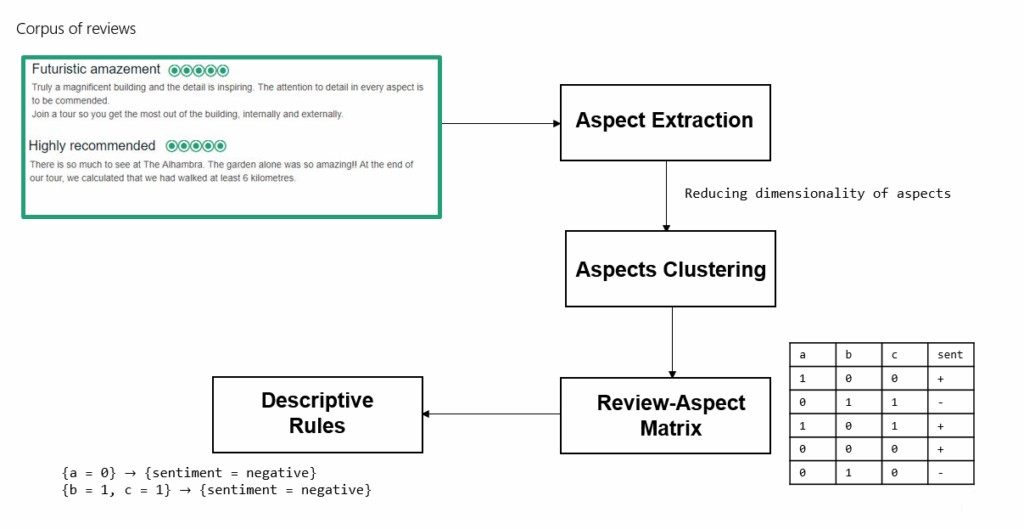
This dataset has been employed for the evaluation of a opinion summarisation methodology which is in review.
Download
Dataset can be downloaded from the repository https://github.com/ari-dasci/OD-TripM
Date
October 2020