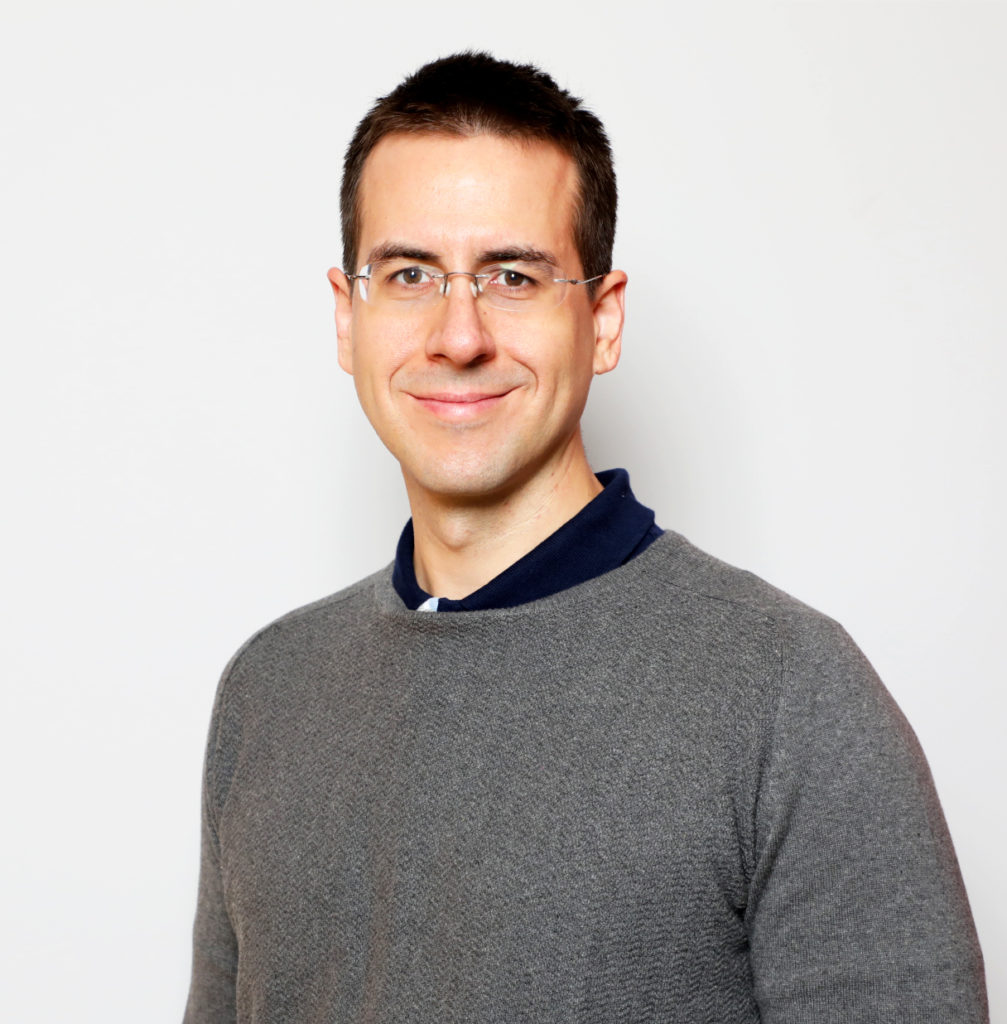
Julián Luengo Martín
Contact
julianlm@decsai.uVS2qKtxvgr.es
Organization
UGRHighly Cited Researcher 2018 - CV -
Total | From 2020: | |
---|---|---|
Citas | Total: 15569 | From 2020: 9141 |
Índice H | Total: 38 | From 2020: 35 |
Índice i10 | Total: 70 | From 2020: 57 |
Papers (147)
Title | Authors | Year |
---|---|---|
SHIELD: A regularization technique for eXplainable Artificial Intelligence | I Sevillano-García, J Luengo, F Herrera. | 2024 |
Combining traditional and spiking neural networks for energy-efficient detection of Eimeria parasites | IX Vázquez, BWD Ayasi, H Seker, J Luengo, J Sedano, AM García-Vico. | 2024 |
Local Attention: Enhancing the Transformer Architecture for Efficient Time Series Forecasting | I Aguilera-Martos, A Herrera-Poyatos, J Luengo, F Herrera. | 2024 |
Local Attention Mechanism: Boosting the Transformer Architecture for Long-Sequence Time Series Forecasting | I Aguilera-Martos, A Herrera-Poyatos, J Luengo, F Herrera. | 2024 |
Developing Big Data Anomaly Dynamic and Static Detection Algorithms: AnomalyDSD Spark Package | D García-Gil, D López, D Argüelles-Martino, J Carrasco, I Aguilera-Martos, .... | 2024 |
X-SHIELD: Regularization for eXplainable Artificial Intelligence | I Sevillano-García, J Luengo, F Herrera. | 2024 |
A Survey on Semi-Supervised Semantic Segmentation | A Peláez-Vegas, P Mesejo, J Luengo. | 2023 |
Multi-step Histogram Based Outlier Scores for Unsupervised Anomaly Detection: ArcelorMittal Engineering Dataset Case of Study | I Aguilera-Martos, M García-Barzana, D García-Gil, J Carrasco, D López, .... | 2023 |
Fusing anomaly detection with false positive mitigation methodology for predictive maintenance under multivariate time series | D López, I Aguilera-Martos, M García-Barzana, F Herrera, D García-Gil, .... | 2023 |
Check for updates Optimizing LIME Explanations Using | I Sevillano-Garcia, J Luengo, F Herrera. | 2023 |
Revisiting Histogram Based Outlier Scores: Strengths and Weaknesses | I Aguilera-Martos, J Luengo, F Herrera. | 2023 |
Optimizing LIME Explanations Using REVEL Metrics | I Sevillano-Garcia, J Luengo, F Herrera. | 2023 |
Low-Impact Feature Reduction regularization term: How to improve Artificial Intelligence with Explainability | I Sevillano-García, J Luengo, F Herrera. | 2023 |
REVEL Framework to Measure Local Linear Explanations for Black‐Box Models: Deep Learning Image Classification Case Study | I Sevillano-García, J Luengo, F Herrera. | 2023 |
Low-Impact Feature Reduction Regularization Term: How to Improve Artificial Intelligence with Explainability. | I Sevillano-García, J Luengo, F Herrera. | 2023 |
The impact of heterogeneous distance functions on missing data imputation and classification performance | MS Santos, PH Abreu, A Fernández, J Luengo, J Santos. | 2022 |
TSFEDL: A Python Library for Time Series Spatio-Temporal Feature Extraction and Prediction using Deep Learning (with Appendices on Detailed Network Architectures and … | I Aguilera-Martos, ÁM García-Vico, J Luengo, S Damas, FJ Melero, .... | 2022 |
TSFEDL: A Python Library for Time Series Spatio-Temporal Feature Extraction and Prediction using Deep Learning | I Aguilera-Martos, ÁM García-Vico, J Luengo, S Damas, FJ Melero, .... | 2022 |
REVEL Framework to measure Local Linear Explanations for black-box models: Deep Learning Image Classification case of study | I Sevillano-García, J Luengo-Martín, F Herrera. | 2022 |
REVEL Framework to Measure Local Linear Explanations for Black-Box Models: Deep Learning Image Classification Case Study | I Sevillano-García, J Luengo, F Herrera. | 2022 |
Multiple instance classification: Bag noise filtering for negative instance noise cleaning | J Luengo, D Sánchez-Tarragó, RC Prati, F Herrera. | 2021 |
A tutorial on the segmentation of metallographic images: Taxonomy, new MetalDAM dataset, deep learning-based ensemble model, experimental analysis and challenges | J Luengo, R Moreno, I Sevillano, D Charte, A Peláez-Vegas, .... | 2021 |
A robust approach for deep neural networks in presence of label noise: relabelling and filtering instances during training | A Gómez-Ríos, J Luengo, F Herrera. | 2021 |
Anomaly Detection in Predictive Maintenance: A New Evaluation Framework for Temporal Unsupervised Anomaly Detection Algorithms | J Carrasco, I Markova, D López, I Aguilera, D García, M García-Barzana, .... | 2021 |
Enhancing instance-level constrained clustering through differential evolution | G González-Almagro, J Luengo, JR Cano, S García. | 2021 |
ME-MEOA/DCC: Multiobjective constrained clustering through decomposition-based memetic elitism | G González-Almagro, A Rosales-Pérez, J Luengo, JR Cano, S García. | 2021 |
3SHACC: Three Stages Hybrid Agglomerative Constrained Clustering | G González-Almagro, JL Suarez, J Luengo, JR Cano, S García. | 2021 |
Similarity-based and Iterative Label Noise Filters for Monotonic Classification | JR Cano, J Luengo, S García. | 2020 |
Reconstrucciones “Resilientes” de la Identidad Profesional del Profesorado: Endoprivatización y Cultura Performativa en Andalucía (España) | J Molina-Pérez, J Luengo. | 2020 |
Big Data: Technologies and Tools | J Luengo, D García-Gil, S Ramírez-Gallego, S García, F Herrera. | 2020 |
Smart Data | J Luengo, D García-Gil, S Ramírez-Gallego, S García, F Herrera. | 2020 |
Final Thoughts: From Big Data to Smart Data | J Luengo, D García-Gil, S Ramírez-Gallego, S García, F Herrera. | 2020 |
Big Data Discretization | J Luengo, D García-Gil, S Ramírez-Gallego, S García, F Herrera. | 2020 |
Imbalanced Data Preprocessing for Big Data | J Luengo, D García-Gil, S Ramírez-Gallego, S García, F Herrera. | 2020 |
Big Data Software | J Luengo, D García-Gil, S Ramírez-Gallego, S García, F Herrera. | 2020 |
Dimensionality Reduction for Big Data | J Luengo, D García-Gil, S Ramírez-Gallego, S García, F Herrera. | 2020 |
Data Reduction for Big Data | J Luengo, D García-Gil, S Ramírez-Gallego, S García, F Herrera. | 2020 |
Big Data Preprocessing: Enabling Smart Data | J Luengo. | 2020 |
Imperfect Big Data | J Luengo, D García-Gil, S Ramírez-Gallego, S García, F Herrera. | 2020 |
DILS: constrained clustering through Dual Iterative Local Search | G González-Almagro, J Luengo, JR Cano, S García. | 2020 |
COVIDGR dataset and COVID-SDNet methodology for predicting COVID-19 based on Chest X-Ray images | S Tabik, A Gómez-Ríos, JL Martín-Rodríguez, I Sevillano-García, .... | 2020 |
Improving constrained clustering via decomposition-based multiobjective optimization with memetic elitism | G González-Almagro, A Rosales-Pérez, J Luengo, JR Cano, S García. | 2020 |
Synthetic Sample Generation for Label Distribution Learning | M González, J Luengo, JR Cano, S García. | 2020 |
Agglomerative Constrained Clustering Through Similarity and Distance Recalculation | G González-Almagro, JL Suarez, J Luengo, JR Cano, S García. | 2020 |
Big data preprocessing | J Luengo, D García-Gil, S Ramírez-Gallego, S García, F Herrera. | 2020 |
Similarity-based and Iterative Label Noise Filters for Monotonic Classification. | JR Cano, J Luengo, S García. | 2020 |
Smartdata: data preprocessing to achieve smart data in R | I Cordón, J Luengo, S García, F Herrera, F Charte. | 2019 |
Label noise filtering techniques to improve monotonic classification | JR Cano, J Luengo, S García. | 2019 |
Towards Highly Accurate Coral Texture Images Classification Using Deep Convolutional Neural Networks and Data Augmentation | A Gómez-Ríos, S Tabik, J Luengo, ASM Shihavuddin, B Krawczyk, .... | 2019 |
Redes Neuronales Convolucionales para Una Clasificacion Precisa de Imágenes de Corales | A Gómez-Rıos, S Tabik, J Luengo, F Herrera, ASM Shihavuddin, .... | 2019 |
Enabling smart data: noise filtering in big data classification | D García-Gil, J Luengo, S García, F Herrera. | 2019 |
Emerging topics and challenges of learning from noisy data in nonstandard classification: a survey beyond binary class noise | RC Prati, J Luengo, F Herrera. | 2019 |
Transforming big data into smart data: An insight on the use of the k‐nearest neighbors algorithm to obtain quality data | I Triguero, D García‐Gil, J Maillo, J Luengo, S García, F Herrera. | 2019 |
Coral species identification with texture or structure images using a two-level classifier based on Convolutional Neural Networks | A Gómez-Ríos, S Tabik, J Luengo, ASM Shihavuddin, F Herrera. | 2019 |
Fast and Scalable Approaches to Accelerate the Fuzzy k Nearest Neighbors Classifier for Big Data | J Maillo, I Triguero, J Luengo, FH S. García. | 2019 |
From Big to Smart Data: Iterative Ensemble Filter for Noise Filtering in Big Data classification | FH Diego Garcia, Francisco Luque-Sánchez, Julián Luengo, Salvador García. | 2019 |
Preprocessing methodology for time series: an industrial world application case study | JA Cortés-Ibáñez, S González, JJ Valle-Alonso, J Luengo, S García, .... | 2019 |
Big Data Preprocessing as the Bridge between Big Data and Smart Data: BigDaPSpark and BigDaPFlink Libraries | D Garcıa-Gil, A Alcalde-Barros, J Luengo, S Garcıa, F Herrera. | 2019 |
Fast and Scalable Approaches to Accelerate the Fuzzy k-Nearest Neighbors Classifier for Big Data | J Maillo, S García, J Luengo, F Herrera, I Triguero. | 2019 |
Big Data Preprocessing as the Bridge between Big Data and Smart Data: BigDaPSpark and BigDaPFlink Libraries. | D García-Gil, A Alcalde-Barros, J Luengo, S García, F Herrera. | 2019 |
CNC-NOS: Class noise cleaning by ensemble filtering and noise scoring | J Luengo, SO Shim, S Alshomrani, A Altalhi, F Herrera. | 2018 |
A preliminary study on Hybrid Spill-Tree Fuzzy k-Nearest Neighbors for big data classification | J Maillo, J Luengo, S García, F Herrera, I Triguero. | 2018 |
A First Study on the Use of Noise Filtering to Clean the Bags in Multi-Instance Classification | J Luengo, D Sánchez-Tarragó, RC Prati, F Herrera. | 2018 |
Un enfoque aproximado para acelerar el algoritmo de clasificacion Fuzzy kNN para Big Data | J Maillo, J Luengo, S Garcıa, F Herrera, I Triguero. | 2018 |
Smart Data: Filtrado de Ruido para Big Data | DJG Gil, J Luengo, SG Gil, F Herrera. | 2018 |
Task Recommendation for Group Users in Public IoT Environments | JS Lee, MH Kim, IY Ko. | 2018 |
KEEL 3.0: an open source software for multi-stage analysis in data mining | I Triguero, S González, JM Moyano, S García López, J Alcalá Fernández, .... | 2017 |
Exact fuzzy k-nearest neighbor classification for big datasets | J Maillo, J Luengo, S García, F Herrera, I Triguero. | 2017 |
A study on the noise label influence in boosting algorithms: AdaBoost, GBM and XGBoost | A Gómez-Ríos, J Luengo, F Herrera. | 2017 |
The noisefiltersr package: label noise preprocessing in R | P Morales, J Luengo, LPF Garcia, AC Lorena, AC de Carvalho, F Herrera. | 2017 |
The NoiseFiltersR Package: Label Noise Preprocessing in R. | P Morales, J Luengo, LPF Garcia, AC Lorena, AC de Carvalho, F Herrera. | 2017 |
Protestando en Twitter: ciudadanía y empoderamiento desde la educación pública= Protesting on Twitter: Citizenship and Empowerment from Public Education | G Saura, JL Muñoz, J Luengo, JM Martos. | 2017 |
The influence of noise on the evolutionary fuzzy systems for subgroup discovery | J Luengo, AM García-Vico, MD Pérez-Godoy, CJ Carmona. | 2016 |
Tutorial on practical tips of the most influential data preprocessing algorithms in data mining | S García, J Luengo, F Herrera. | 2016 |
Big data preprocessing: methods and prospects | S García, S Ramírez-Gallego, J Luengo, JM Benítez, F Herrera. | 2016 |
INFFC: an iterative class noise filter based on the fusion of classifiers with noise sensitivity control | JA Sáez, M Galar, J Luengo, F Herrera. | 2016 |
Evaluating the classifier behavior with noisy data considering performance and robustness: The equalized loss of accuracy measure | JA Sáez, J Luengo, F Herrera. | 2016 |
From big data to smart data with the k-nearest neighbours algorithm | I Triguero, J Maillo, J Luengo, S García, F Herrera. | 2016 |
A first study on the use of boosting for class noise reparation | PM Álvarez, J Luengo, F Herrera. | 2016 |
Comparison of KEEL versus open source Data Mining tools: Knime and Weka software | I Triguero, S González, JM Moyano, S García, J Alcala-Fdez, J Luengo, .... | 2016 |
Mixtures of Polynomials for Regression Problems | JC Luengo, R Rumi. | 2016 |
Package ‘NoiseFiltersR’ | P Morales, J Luengo, LPF Garcia, AC Lorena, AC de Carvalho, F Herrera, .... | 2016 |
A first approach in the class noise filtering approaches for fuzzy subgroup discovery | CJ Carmona, J Luengo. | 2015 |
SMOTE–IPF: Addressing the noisy and borderline examples problem in imbalanced classification by a re-sampling method with filtering | JA Sáez, J Luengo, J Stefanowski, F Herrera. | 2015 |
Using the One-vs-One decomposition to improve the performance of class noise filters via an aggregation strategy in multi-class classification problems | LPF Garcia, JA Sáez, J Luengo, AC Lorena, AC de Carvalho, F Herrera. | 2015 |
An automatic extraction method of the domains of competence for learning classifiers using data complexity measures | J Luengo, F Herrera. | 2015 |
Naive Bayes classifier with mixtures of polynomials | J Luengo, R Rumi. | 2015 |
Naive Bayes Classifier with Mixtures of Polynomials. | J Luengo, R Rumí. | 2015 |
Intelligent Systems Reference Library 72 Data Preprocessing in Data Mining | S García, J Luengo, F Herrera. | 2015 |
Data preprocessing in data mining, Springer International Publishing | S García, J Luengo, F Herrera. | 2015 |
Data Preprocessing in Data Mining | S García, J Luengo, F Herrera. | 2014 |
Analyzing the presence of noise in multi-class problems: alleviating its influence with the one-vs-one decomposition | JA Sáez, M Galar, J Luengo, F Herrera. | 2014 |
On the characterization of noise filters for self-training semi-supervised in nearest neighbor classification | I Triguero, JA Sáez, J Luengo, S García, F Herrera. | 2014 |
Statistical computation of feature weighting schemes through data estimation for nearest neighbor classifiers | JA Sáez, J Derrac, J Luengo, F Herrera. | 2014 |
Managing borderline and noisy examples in imbalanced classification by combining SMOTE with ensemble filtering | JA Sáez, J Luengo, J Stefanowski, F Herrera. | 2014 |
Big data analytics | B Analytics. | 2014 |
Las políticas y legislación españolas de formación profesional: las consecuencias de la aprobación de la Ley de Cualificaciones y de la Formación Profesional y de la Ley de … | M Jiménez, J Luengo, D Sevilla. | 2014 |
Improving the behavior of the nearest neighbor classifier against noisy data with feature weighting schemes | JA Sáez, J Derrac, J Luengo, F Herrera. | 2014 |
4. Evaluation measures of the behavior of classifiers with noisy data | JA Sáez, J Luengo, F Herrera. | 2014 |
Journal: Intelligent Systems Reference Library Data Preprocessing in Data Mining, 2014, p. 1-17 | S García, J Luengo, F Herrera. | 2014 |
Predicting noise filtering efficacy with data complexity measures for nearest neighbor classification | JA SáEz, JN Luengo, F Herrera. | 2013 |
Tackling the problem of classification with noisy data using Multiple Classifier Systems: Analysis of the performance and robustness | JA SáEz, M Galar, JN Luengo, F Herrera. | 2013 |
An Experimental Case of Study on the Behavior of Multiple Classifier Systems with Class Noise Datasets | JA Sáez, M Galar, J Luengo, F Herrera. | 2013 |
Dinámicas endógenas de privatización en la educación. La cultura de la performatividad | J Luengo, G Saura. | 2013 |
Nuevas formas de mercantilizar la educación | J Luengo, G Saura. | 2013 |
An analysis on the use of pre-processing methods in evolutionary fuzzy systems for subgroup discovery | CJ Carmona, J Luengo, P González, MJ Del Jesus. | 2012 |
A Preliminary Study on Selecting the Optimal Cut Points in Discretization by Evolutionary Algorithms. | S García, V López, J Luengo, CJ Carmona, F Herrera. | 2012 |
A preliminary study on missing data imputation in evolutionary fuzzy systems of subgroup discovery | CJ Carmona, J Luengo, P González, MJ del Jesus. | 2012 |
A survey of discretization techniques: Taxonomy and empirical analysis in supervised learning | S Garcia, J Luengo, JA Sáez, V Lopez, F Herrera. | 2012 |
On the choice of the best imputation methods for missing values considering three groups of classification methods | J Luengo, S García, F Herrera. | 2012 |
Missing data imputation for fuzzy rule-based classification systems | J Luengo, JA Sáez, F Herrera. | 2012 |
Shared domains of competence of approximate learning models using measures of separability of classes | J Luengo, F Herrera. | 2012 |
A first study on decomposition strategies with data with class noise using decision trees | JA Sáez, M Galar, J Luengo, F Herrera. | 2012 |
On the suitability of fuzzy rule-based classification systems with noisy data | J Saez, J Luengo, F Herrera. | 2012 |
La gestión de resultado como mecanismo endógeno de privatización en educación | J Luengo, G Saura. | 2012 |
Evolutionary selection of hyperrectangles in nested generalized exemplar learning | S García, J Derrac, J Luengo, CJ Carmona, F Herrera. | 2011 |
Keel data-mining software tool: data set repository, integration of algorithms and experimental analysis framework. | J Alcalá-Fdez, A Fernández, J Luengo, J Derrac, S García, L Sánchez, .... | 2011 |
Addressing data complexity for imbalanced data sets: analysis of SMOTE-based oversampling and evolutionary undersampling | J Luengo, A Fernández, S García, F Herrera. | 2011 |
Fuzzy rule based classification systems versus crisp robust learners trained in presence of class noise's effects: a case of study | JA Sáez, J Luengo, F Herrera. | 2011 |
Using KEEL software as a educational tool: A case of study teaching data mining | J Derrac, J Luengo, J Alcala-Fdez, A Fernandez, S Garcia. | 2011 |
Imputation of Missing Values | J Luengo, S Garcıa, F Herrera. | 2011 |
Soft Computing Techniques in Data Mining. | J Alcalá-Fdez, F Herrera, H Ishibuchi, Y Kaisho, Y Nojima, BC Chien, .... | 2011 |
KEEL data-mining software tool: Data set repository, integration of algorithms and experimental analysis framework | J Alcala-Fdez, A Fernández, J Luengo, J Derrac, S García, L Sánchez, .... | 2011 |
Keel data-mining software tool: data set repository, integration of algorithms and experimental analysis framework. J Mult-Valued Log Soft Comput 17: 255–287 | J Alcalá-Fdez, A Fernandez, J Luengo, J Derrac, S García, L Sánchez, .... | 2011 |
867 L. Sánchez and F. Herrera, KEEL Data-Mining Software Tool: 868 Data Set Repository, Integration of Algorithms and Experi- 869 mental Analysis Framework | J Alcalá-Fdez, A Fernandez, J Luengo, J Derrac, S Garcıa. | 2011 |
A software tool to assess evolutionary algorithms for data mining problems | J Alcala-Fdez, A Fernandez, J Luengo, J Derrac, S Garcia, L Sanchez, .... | 2011 |
Garcí*** a, S., Sánchez, L. and Herrera, F., KEEL data-mining software tool: data set repository, integration of algorithms and experimental analysis framework | J Alcalá-Fdez, A Fernández, J Luengo, J Derrac. | 2011 |
Advanced nonparametric tests for multiple comparisons in the design of experiments in computational intelligence and data mining: Experimental analysis of power | S García, A Fernández, J Luengo, F Herrera. | 2010 |
Genetics-based machine learning for rule induction: state of the art, taxonomy, and comparative study | A Fernández, S García, J Luengo, E Bernadó-Mansilla, F Herrera. | 2010 |
A study on the use of imputation methods for experimentation with Radial Basis Function Network classifiers handling missing attribute values: The good synergy between RBFNs … | J Luengo, S García, F Herrera. | 2010 |
Domains of competence of fuzzy rule based classification systems with data complexity measures: A case of study using a fuzzy hybrid genetic based machine learning method | J Luengo, F Herrera. | 2010 |
Geneticsbased machine learning for rule induction: Taxonomy, experimental study and state of the art | A Fernández, S García, J Luengo, E Bernadó-Mansilla, F Herrera. | 2010 |
A first study on the noise impact in classes for fuzzy rule based classification systems | JA Sáez, J Luengo, F Herrera. | 2010 |
An extraction method for the characterization of the Fuzzy Rule Based Classification Systems' behavior using data complexity measures: A case of study with FH-GBML | J Luengo, F Herrera. | 2010 |
Genetics-based machine learning for rule induction: Taxonomy, experimental study and state of the art | A Fernández, S García, J Luengo, E Bernadó-Mansilla, F Herrera. | 2010 |
Determinando Automáticamente los Dominios de Competencia de un Sistema de Clasificación Basado en Reglas Difusas: Un Caso de Estudio con FH-GBML | J Luengo, F Herrera. | 2010 |
Obtención de los dominios de competencia de C4. 5 por medio de medidas de separabilidad de clases | J Luengo, F Herrera. | 2010 |
Soft computing based learning and data analysis: missing values and data complexity | J Luengo Martín. | 2010 |
Análisis de impacto del ruido en clases y atributos para Sistemas de Clasificación Basados en Reglas Difusas | JA Sáez, J Luengo, F Herrera. | 2010 |
A study of statistical techniques and performance measures for genetics-based machine learning: accuracy and interpretability | S García, A Fernández, J Luengo, F Herrera. | 2009 |
A study on the use of statistical tests for experimentation with neural networks: Analysis of parametric test conditions and non-parametric tests | J Luengo, S García, F Herrera. | 2009 |
A first approach to nearest hyperrectangle selection by evolutionary algorithms | S García, J Derrac, J Luengo, F Herrera. | 2009 |
Addressing data-complexity for imbalanced data-sets: A preliminary study on the use of preprocessing for c4. 5 | J Luengo, A Fernández, S García, F Herrera. | 2009 |
Implementation and integration of algorithms into the KEEL data-mining software tool | A Fernández, J Luengo, J Derrac, J Alcalá-Fdez, F Herrera. | 2009 |
On the use of Measures of Separability of Classes to Characterise the Domains of Competence of a Fuzzy Rule Based Classification System. | J Luengo, F Herrera. | 2009 |
Domains of competence of artificial neural networks using measures of separability of classes | J Luengo, F Herrera. | 2009 |
Social and educational exclusion as failures. Framework for their understanding and research | M Jiménez, JJ Luengo, J Taberner. | 2009 |
Competence-based approach in the development teacher training policy. Interview with Claude Lessard | J Luengo, A Luzón, M Torres. | 2008 |
A study on the use of statistical tests for experimentation with neural networks | J Luengo, S García, F Herrera. | 2007 |
Our experience in primary hyperaldosteronism | S Gallego, A Covarsí, J Luengo, P González, MA Suarez, R Novillo. | 2007 |
Nuestra experiencia en el hiperaldosteronismo primario | S Gallego, A Covarsí, J Luengo, P González, MA Suarez, R Novillo. | 2007 |
From Centralism to Decentralization. | J Luengo, D Sevilla, M Torres. | 2005 |
La educación como objeto de conocimiento. El concepto de educación | J Luengo. | 2004 |
La educación como hecho | J Luengo. | 2004 |
Revisión bibliográfica de disfunción craneomandibular del aņo 2000 | O Rustullet Maņé, S Fradera, ÓJ Villalba Moreno, M Castellsagué, .... | 2001 |
Disfunción craneomandibular. Revisión bibliográfica del aņo 1999 | M Castellsagué, J Masdevall, O Rustullet Maņé, S Fradera, J Sabriā Rius, .... | 2000 |
Disfunción craneomandibular. Revisión bibliográfica del año 1999 | M Castellsagué, J Masdevall, O Rustullet, S Fradera, JS Rius, O Villalba, .... | 2000 |
Disfunción craneomandibular. Revisión bibliográfica del año 1998 | M Castellsagué, S Fradera, J Masdevall, J Pujol, O Rustullet, .... | 1999 |
Disfunción craneomandibular. Revisión bibliográfica del año 1997 | M Castellsagué, J Masdevall, A Revilla, JS Rius, S Fradera, J Pujol, .... | 1998 |
Descargando datos de la publicación