Autism Spectrum Disorder
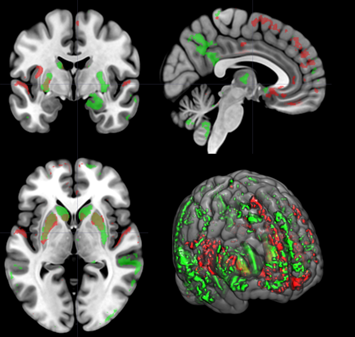
Machine Learning Techniques applied to the NeuroScience of the Autism Spectrum Disorder
Nowadays, structural and functional imaging technologies in neuroscience are providing a high volume of information, where machine learning-based approaches are qualified to provide greater insight into psychiatric disorders, such as the Autism Spectrum Condition (ASC). Beyond the standard data mining-based systems, and given the massive amounts of computational power available, researchers are in the starting point to overtake the classical psychiatric paradigms of the last fifty years, proposing new measures of variable importance and deep learning architectures to reveal hidden features in several neurological disorders. The main outstanding results are:
1. Classification methods (see paper at https://www.ncbi.nlm.nih.gov/pmc/articles/PMC4047979/ ) based on hybrid architectures within the CAD systems using feature selection and extraction and statistical classification methods. The resulting image patterns from MRI acquisitions are subjected to classification in order to demonstrate the existence of an endophenotype of ASC
2. We have developed a technique called Significance Weighted Principal Component Analysis (SWPCA) to reduce the undesired intensity variance due to acquisition site and to increase the statistical power in detecting group differences (see paper at https://www.ncbi.nlm.nih.gov/pubmed/27774713 ) under classical validation schemes which, in this context, globally characterize this highly heterogeneous condition by neuroimaging with difficulty.
3. Novel and simpler error estimators with upper bounds, like those based on resubstitution error estimate, to provide relevant information on this condition (see paper at https://www.worldscientific.com/doi/10.1142/S0129065718500582 ). In this sense, a four-class classification problem (sex and condition) is specified, with theoretical restrictions in terms of the classifier complexity and feature space dimension, revealed how autism is modulated by biological sex using a low-dimensional feature space extracted from voxel based morphometry (VBM). In addition, a spatial overlap analysis on reference maps partially corroborates predictions of the “extreme male brain” theory of autism, in sexual dimorphic areas.
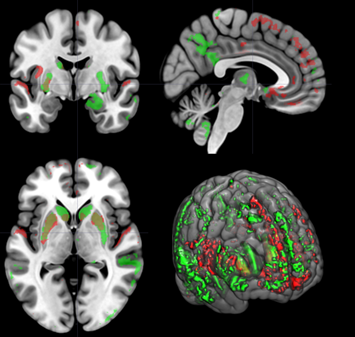
This way, neuroimaging studies have reported structural and physiological differences that could help understand the causes and development of Autism Spectrum Disorder (ASD). Finally, all these machine learning based techniques have the potential to yield the in-vivo assessment of brain functional/structural parameters in several neurological disorders. Relevant links that highlight the outstanding results are found in:Finalmente, todas estas técnicas basadas en aprendizaje máquina presentan la potencialidad de proporcionar una evaluación in-vivo de parámetros cerebrales funcionales/estructurales en numerosos desórdenes neurológicos. Enlaces de relevancia de los resultados destacados pueden encontrase en: https://github.com/SiPBA/swpca
Period
May 2007 – Present
Researchers
Juan Manuel Górriz Sáez, Javier Ramírez Pérez de Inestrosa, Fermín Segovia Román, Francisco J. Martínez Murcia.
Collaborators: Prof. John Suckling, University of Cambridge, UK, Prof. Simon Baron Cohen, University of Cambridge, UK, Prof. Michael Lombardo, University of Cyprus, CY., Prof. Meng-Chuan Lai, University of Toronto, CAN.