Schizophrenia
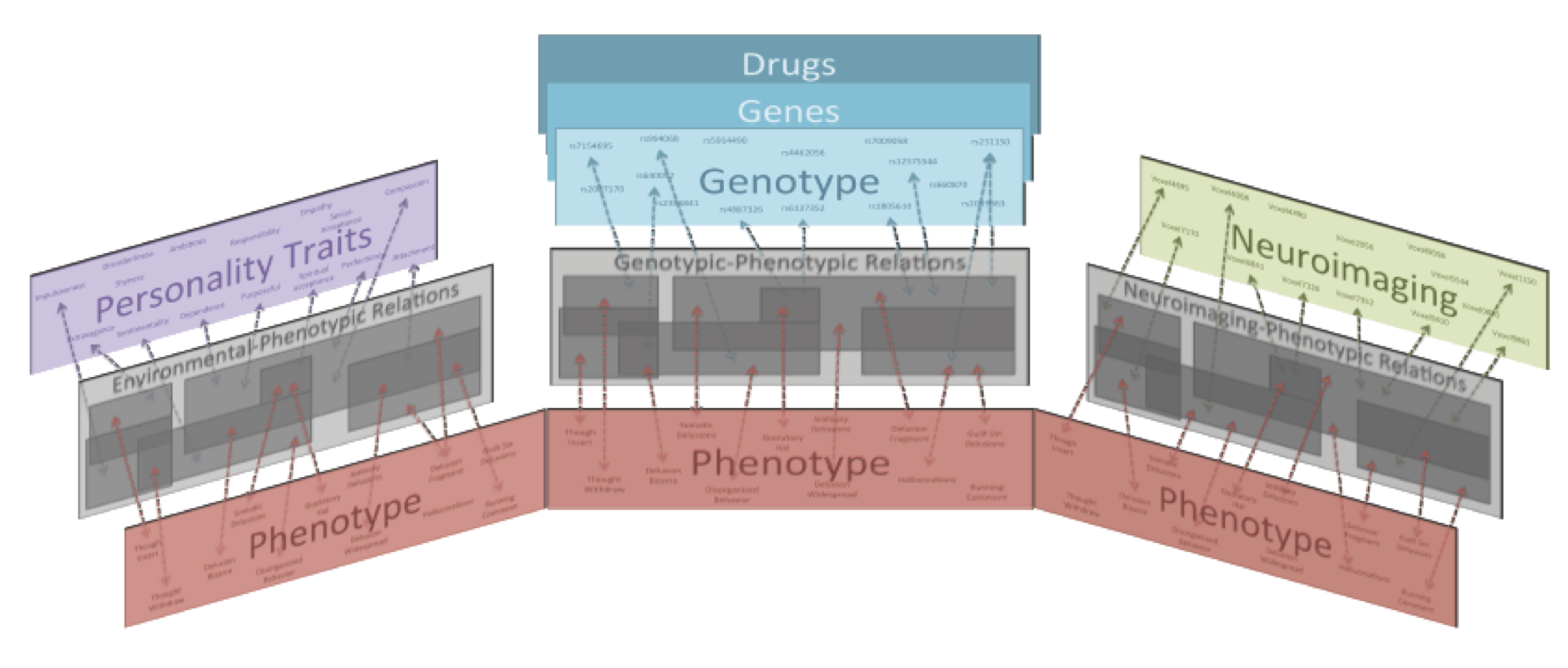
Machine learning for schizophrenia study
Schizophrenia not a single disease but multiple genetically distinct disorders.
Most human complex diseases and disorders result from a complex interplay between multiple genetic and environmental factors. It is known that hundreds or thousands of genetic variants (SNPs) interact with one another in complex ways, and consequently display a multifaceted genetic architecture that may influence complex diseases. The genetic architecture of heritable diseases refers to the number, frequency, and effect sizes of genetic risk alleles and the way they are organized into genotypic networks.
Schizophrenia is a complex mental disease that affect 1% of the population. In Spain there are more than 500.000 known cases. However, thought twin and family studies of schizophrenia indicate that the risk of suffering the disease is highly heritable (81%) only 25% of the variability has been explained by specific genetic variants (SNPs) identified in genome-wide association studies (GWAS). The frequent failure to account for most of the heritability of complex disorders has been called the “missing” or “hidden” heritability problem. More over these genetic association studies of complex disorders have largely produced weak and inconsistent or unreplicable findings that make very difficult bridging the gaps between bench and bedside.
We have developed a new deep unsupervised and data-driven machine learning method, PGMRA http://phop.ugr.es/fenogeno that combines Model-based, Consensus, Fuzzy, Possibilistic, Relational, Optimization, and Conceptual clustering techniques into a single method to discover interesting groups (SNPs sets, phenotypic sets, etc..) defined in distinct knowledge domains such as: phenotype, genotype (SNPs), images, TCI inventories, and interesting relations between those groups (clusters). The most interesting characteristics of the method are:
- the grouping strategy does not use previous knowledge about other studies or genomic features and does not consider the status of the subjects (ill or control) in the data set to identify groups of SNP or phenotype sets (i.e. unsupervised learning);
- subjects, SNPs and/or phenotype features can belong to more than one relation of groups;
- SNPs within an SNP set can be located anywhere in the genome;
- the dimensionality of the phenotype features is not reduced (as would be the case with Principal Component Analysis or similar approaches) because, in phenomics, important features are a priori not known;
- there is no a predefined number of SNP sets and/or phenotype sets and/or relations among them; many-to-many relations among SNP and phenotype sets are identified in an unbiased fashion without considering subject’s disease status (e.g. cases, controls);
- the risk of a disease is estimated in an unbiased fashion by incorporating a posteriori the subject status within each relation, weighing the frequency of each type of status (e.g. cases, relatives, controls) and mapping it into a predictive risk surface.
Moreover, PGMRA can estimate the statistical significance of interactions of SNPs and SNPs sets associated with the disease In sum, PGMRA provides a quick snapshot of different domain knwoledge in an interpretable fashion (https://www.ncbi.nlm.nih.gov/pubmed/23761451)
Outstanding results:
- Applying our method we found that the Schizophrenias could be divided into at least 8 syndromes and replicated the results in three different populations covering all hidden heredability. Our work in the Schizophrenias has already been frequently cited as a ground-breaking resolution of the missing heritability problem and suggested to be applied in other mental and physical disorders beyond the case-control stratification. Indeed, the applied methodology is well aligned with the scope of personalized/precision medicine as understood in the context of cancer diagnosis and treatment, as well as with the guiding principles of RDoC (https://ajp.psychiatryonline.org/doi/full/10.1176/appi.ajp.2014.14040435?url_ver=Z39.88-2003&rfr_id=ori:rid:crossref.org&rfr_dat=cr_pub%3dpubmed).
- We have also found that the genotypic-phenotypic architecture of personality also reduces the gap in heritability estimated from twin studies (50%) and that explained by GWAS. These same personality profiles are predictive of schizophrenia and other psychopathology. Currently we are working on cancer, autisms and depression. (https://www.nature.com/articles/s41380-018-0263-6), (https://www.nature.com/articles/s41380-018-0263-6#MOESM1)
Info and contact: {zwir,delval} at ugr.es
Period
Feb 2015 – Present
Researchers
Igor Zwir, Coral del Val,Rocío Romero-Zaliz, (Andalusian Research Institute on Data Science and Computational Intelligence), Javier Arnedo Fernandez, Alberto Mesa, Claude Robert Cloninger (Department of Psychiatry, Washington University School of Medicine, St. Louis, MO, USA), Terho Lehtimäki (Fimlab Laboratories, Department of Clinical Chemistry, Faculty of Medicine and Life Sciences, Finnish Cardiovascular Research Center-Tampere, University of Tampere, Tampere, Finland)